About
PRECISE provides effective automated solutions to enable efficient, intelligent, and widespread real-time Energy Management (EM) from the consumer side. The increasing use of non-dispatchable Renewable Energy Sources (RES) requires that the load-generation balance is no longer exclusively addressed in a centralized way and driven by rigid demand. Consumers are required to assume an active role and make efficient use of their demand flexibility, as well as of other energy resources they may own, including distributed generation, stationary storage, and electric vehicles. PRECISE models allow the widespread use of local Automated Energy Management (AEM), empowering consumers to reduce their energy bills while ensuring that their needs and preferences are met. This is done by adapting the system’s decisions to different contexts and users’ behaviors, as well as to their evolution over time. In this way, a significant part of the consumers’ flexibility potential can be gathered and used for their own benefit and also for the benefit of the whole system through different demand response schemes.
This is targeted through the conception, development, and implementation of models, methods, and tools able to represent the consumers’ needs and preferences regarding energy use, as well as managers’ and experts’ knowledge regarding EM. PRECISE overcomes current systems limitations, namely the inability to adapt themselves over time to different contexts and types of users, to the evolution of the devices and systems they monitor and act on, and to consider all the data, information, and knowledge they can access. The main goal is to enable the systems and services (S&S) that support consumers in their EM to be used in an automated intelligent way according to the consumers’ needs and preferences. PRECISE advancements enable the targeted S&S to perform automated actions, based on their internal conclusions, and autonomously learn over time while operating in their environment. PRECISE departs from the current Artificial Intelligence state of the art and goes further conceiving, developing, and implementing models and methods that can autonomously operate in real-time, in a continuous way, making use of the multiple data sources, information, and knowledge, while ensuring the consumers, managers, and experts trust on their decisions and actions. To accomplish that, PRECISE proposes Automated Machine Learning (AML) models able to autonomously learn over time and explainable models that allow providing the users with understandable explanations regarding their decisions. Being able to provide users with adequate explanations regarding their behavior and their automated learning, they gain the users’ trust to act in automatic mode as their default operating mode.
To reduce the gap between human knowledge and data-driven approaches, while still profiting from their high efficiency, PRECISE complements the use of Machine Learning (ML) methods with semantic approaches. Semantics makes the systems able to interpret automatically the data, information, and knowledge they receive and to emulate human (e.g., consumers, building managers) decisions and actions. Combining the use of data-driven and knowledge-based approaches, PRECISE makes use of the best advantages of each of those approaches for EM automation. AML brings its efficiency to cope with the real-time requirements and knowledge base (KB) approaches ensure that contexts overlooked by the ML approaches are adequately addressed, according to the consumers’ and managers’ will and needs and based on the experts’ knowledge.
Start Date: March 15th, 2021
Duration: 36 months
End Date: March 14th, 2024
Graphical Abstract
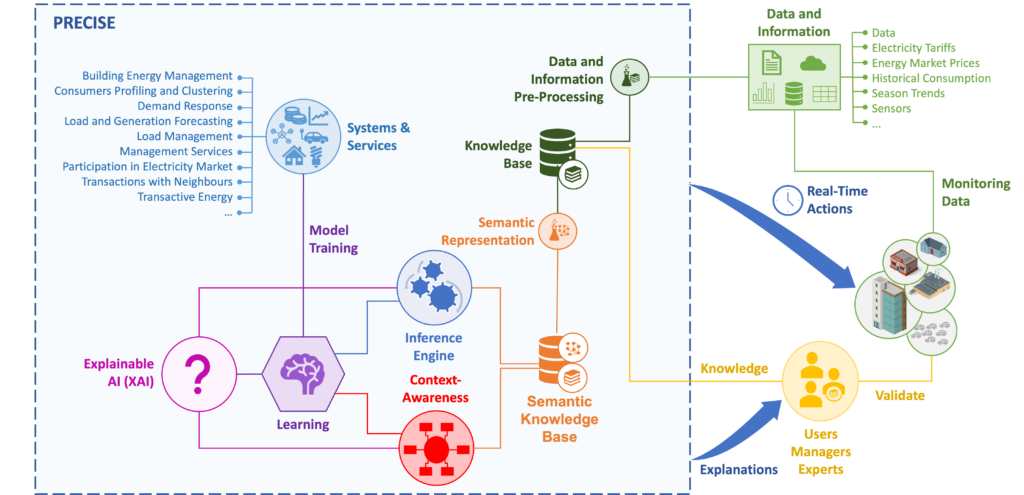
Work Packages (WP)
WP1 Organization and Management
WP1 will ensure the effective coordination of the project, ensuring that the project objectives are achieved within the budget and the time schedule using the available resources effectively. Zita Vale, the PI, will ensure the proper communication and coordination among the involved researchers and facilitate the project activities. She will coordinate periodical meetings and ensure compliance with legal, ethical, and privacy concerns.
WP2 Semantic Knowledge Model
WP2 will conceive and develop the knowledge model to support the PRECISE project, namely in WP3, WP4, and WP5. The goals of WP2 are to develop ontologies able to support the explanation of models and results (to be used in WP3), as well as being capable of describing models that will help in the automated contextual learning (to be used in WP4), and considering the description of cyber-physical infrastructures domain knowledge (for WP5).
WP3 Explainable AI Models
WP3 analyses, conceives, and implements explainable AI (XAI) models that will support the generation of explanations and contribute to the AML of the project solutions. The main objectives are: i) to develop an explanation model library with the necessary flexibility and scalability to allow adding explanations to new and existing AI-based S&S; and ii) providing explanations adapted to different contexts and types of users, namely in terms of the most appropriate language and granularity level. This allows increasing the value of the explanations and the understanding of the S&S by their users and by experts, which is used as the basis for trust. This will also allow, when detecting an anomaly in the used models, to understand why it happened and to make the necessary adjustments. These models will be added to the semantic models from WP2, completing the KB of the systems with the knowledge to be used for their explainability features.
WP4 Automated Multi-Source Contextual Learning
WP4 focuses on the development of the AML models to support the PRECISE project, namely in WP5 and WP6. The main purpose is to test and analyze the obtained results from the considered S&S in order to automatically detect anomalies in real-time. For the anomalies identification, the semantic knowledge model (WP2), the explanations provided by explaining models (WP3), and the detection of the contextual conditions, will be considered. WP4 will benefit from its integration with the cyber-physical infrastructure (WP5), and with the validation process (WP6), in order to check if the automated contextual-learning models are performing adequately.
WP5 Cyber-Physical Infrastructure and Integrated PRECISE Solution
WP5 will build the project solution through the integration of the models developed in WP2, WP3, and WP4. WP5 will also prepare the cyber-physical infrastructure to support the PRECISE proof of concept. The cyber-physical infrastructure based on an original PI vision and built along the years by the GECAD PES team will be improved and adapted to accommodate the integration of the PRECISE solution, enabling the validation of the project solution as a whole. The validation will thus be done not only via simulation studies but also through the impact on actual physical energy resources. This infrastructure will also enable the project solution to be fed by real data from multiple sources in real-time (e.g., locally monitored data and third-party data transmitted in real-time to GECAD servers).
WP6 Experimental Results and Validation
WP6 comprises the experimental results and validation of the solutions developed by the project. The testing and validation process runs throughout the project in an iterative way: models developed in WP2, WP3, and WP4 are validated in WP6, in an independent manner and also through the evaluation of results considering models integration in the cyber-physical infrastructure (WP5). The feedback from the validation is returned to the corresponding tasks for improvement and refinement of the models.
WP7 Communication and Dissemination
WP7 is devoted to the project communication and dissemination activities creating visibility of the project objectives and of the achieved results. WP7 will ensure that the knowledge and results created in the project are disseminated to the appropriate target audiences at a national and international level (scientific community, industry, the general public, and other relevant stakeholders).
Timeline
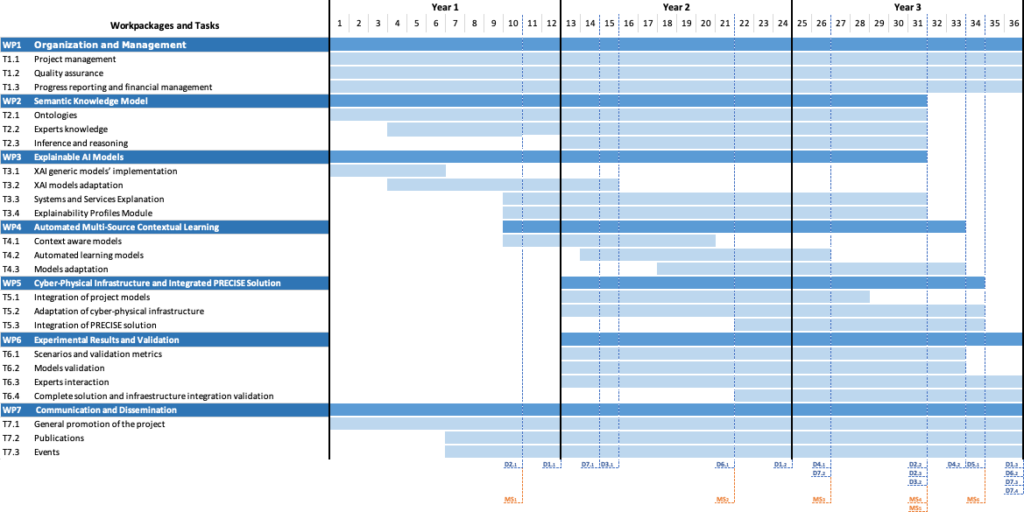
Deliverables and Milestones
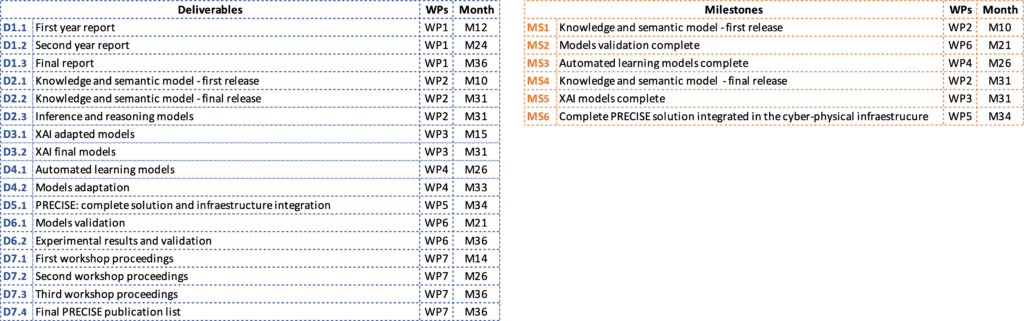
